Shandong Energy Group is a large energy corporation whose business covers mining, high-end chemical products, electric power, new energy materials, high-end equipment manufacturing, and modern logistics and trade. It is China's 3rd largest coal producer and a global leader in smart coal mines. Nine of its mines are national-level showcases for smart coal mines.
Utilizing Huawei Cloud Pangu models, Shandong Energy Group has established a corporate AI training center, and applied the Pangu Mining Model to nine major coal mine processes, including mining, drivage, equipment control, transportation, ventilation, and separation. The Pangu Mining Model integrates the capabilities of four foundation models: computer vision, GNN, multimodal, and NLP. With all-scenario AI applications used at scale, Shandong Energy has reduced its underground workforce while running its coal mines just as efficient and safe.
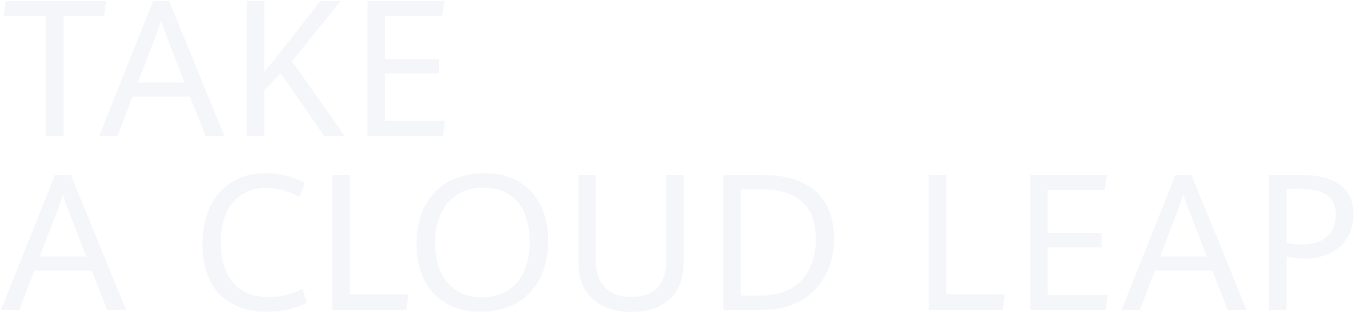
Better AI Models
High training efficiency
Massive data processing capacity
Good generalization
High sample screening efficiency
High accuracy
Leveraging cloud-edge synergy, data is streamlined between the corporate central cloud and the edge (i.e. coal mines). The model excels in continuous learning and few-shot learning, allowing it to achieve high accuracy with limited training data. The model is trained at the Xinglongzhuang Coal Mine, Shandong Energy's smart coal mine showcase, and can then be quickly replicated across its other 70+ mines.
The model, trained on over 1 billion images and 100 TB of video data using unsupervised learning, delivers extraordinary visual representation and recognition performance.
Compared with small models, large models offer better generalization performance. The pre-trained Pangu model can be quickly adapted to new tasks at new coal mines and achieve passable accuracy by generalizing over new data. There is no need to train new models from scratch.
The Pangu model can efficiently obtain defect samples for brand new task scenarios. Compared with traditional methods, the Pangu model cuts the data labeling workload by 85%.
Task-specific models can be quickly trained for various tasks, covering production, safety monitoring, and decision-making, based on the principle of "anything unexpected is abnormal". With few-shot learning, these models achieve 10% higher accuracy than conventional models.
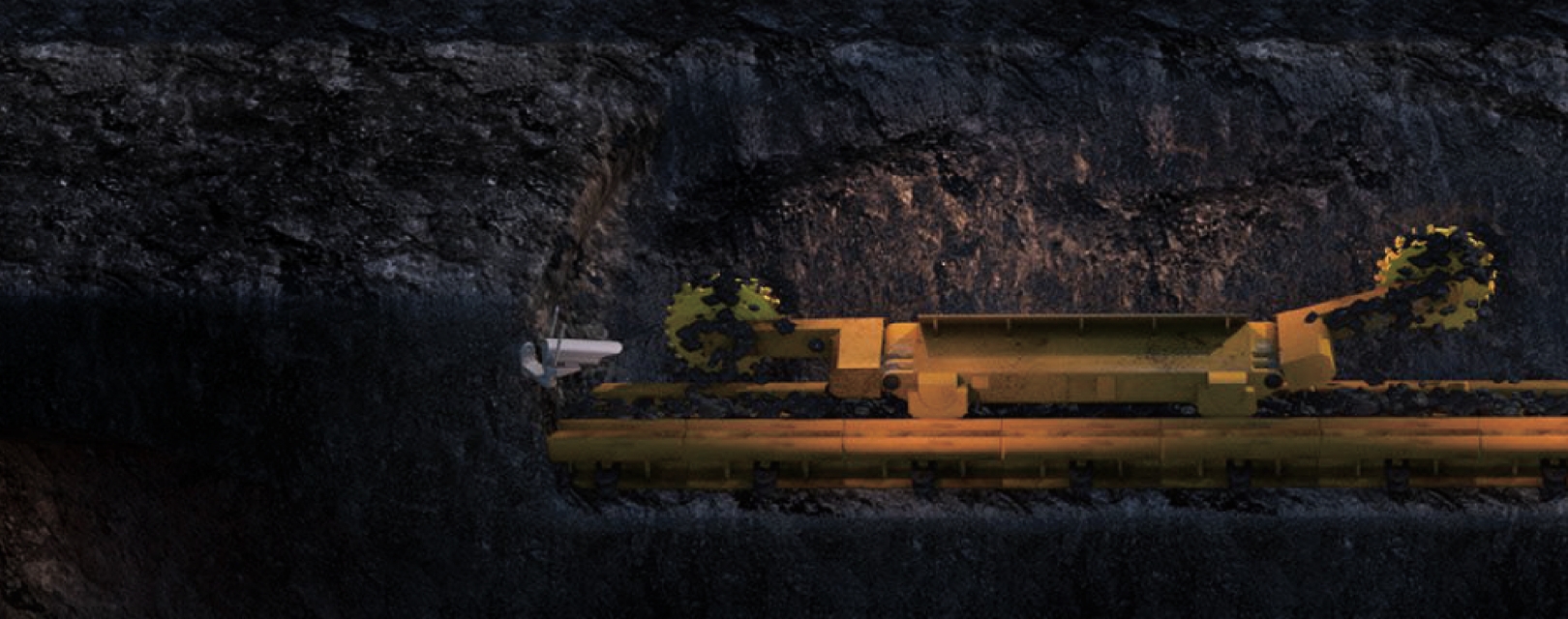
Higher Productivity
In the past, human experts set the input parameters for processes like coal separation and coking coal blending based on their own experience. Now, by modeling real-world production data, the Pangu model accurately predicts outputs based on input parameters, and optimizes these parameters to balance quality and cost, thus maximizing productivity and benefits.
In the case of coal separation, the Pangu GNN model was used to build models that predict the outputs of heavy-media separation and ash content. Parameters are optimized for relevant processes, such as the cyclone. The density of the coal separation media and the inlet pressure can be automatically adjusted based on the ash content of the cleaned coal. The ash content of cleaned coal is stabilized and the yield of cleaned coal is increased by 0.1% to 0.2%. This means a coal mine the size of Jining No. 2 (a coal mine operated by Shandong Energy) is able to increase its production of cleaned coal by 8,000 tons annually. Replicating this solution, coal mines in China could increase their annual production of cleaned coal by an average of 2,000 tons per mine.
In the case of coking coal blending, graph neural network techniques are used to train a coal blending optimization model, which helps accelerate coal blending time from 1 to 2 days to mere minutes for the coking process.
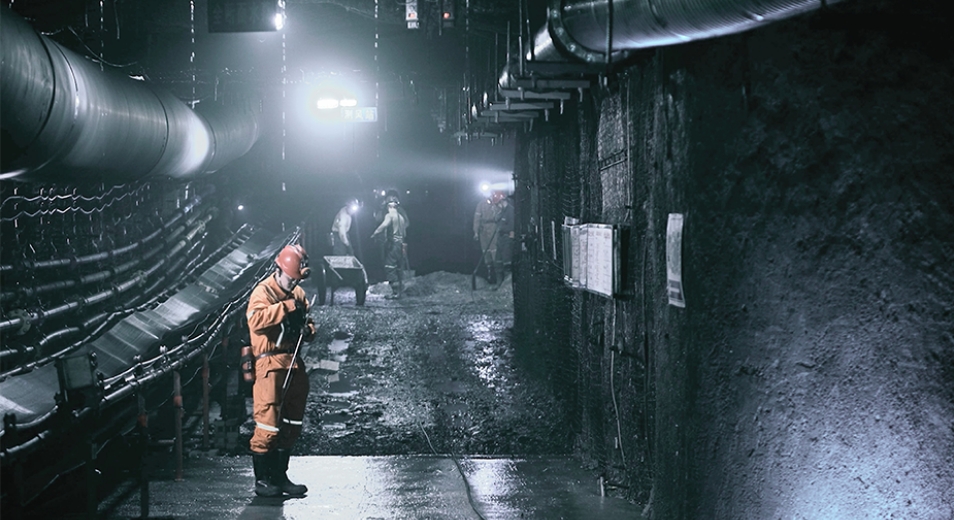
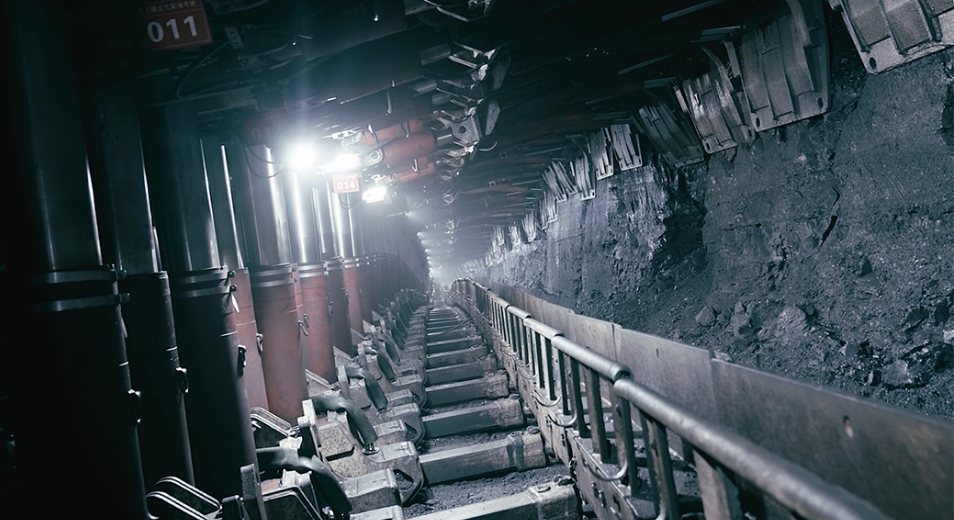
Safer Coal Mines
The Pangu Mining Model powers intelligent video surveillance and inspection in harsh underground coal mine environments. Human inspection is now required only weekly, reducing labor costs and improving safety.
According to results from the Xinglongzhuang Coal Mine Project Phase I, the model achieved over 90% accuracy in detecting human presence in hazardous areas, 10% more accurate than conventional small models. This helps prevent safety hazards from progressing into accidents by issuing prompt warnings. Prompt and accurate warnings on non-compliance worker behavior also help to improve workers' safety awareness.
Drilling depth is a key parameter in anti-burst and pressure relief projects. Lilou Coal Mine in west Shandong used the Pangu Mining Model to monitor this parameter. Dedicated cameras monitor construction in real-time. Videos are uploaded and analyzed by AI in real time. When drilling depth is insufficient, audio-visual alarms are generated promptly. The model also supports functions like construction plan management, video recognition result query, drilling depth verification, and drilling hole counting, enabling prompt and accurate oversight and inspection, while cutting human labor costs by 80%.